
The Scene
The big news — albeit somewhat old news — is that large language models can work with small data.
And that could be game-changing for journalism.
I spent a weekend building some custom bots to explore how newsrooms could leverage generative AI to improve their work. TL;DR: I think there’s a lot of there there.
To recap: LLMs are great at manipulating language — parsing questions, generating human-like prose, and engaging in conversation. But they do poorly at distinguishing fact from fiction — even if they’re improving at an incredible pace — which makes them ill-suited to creating content that’s meant to be accurate, a.k.a journalism. One reason is that they’ve been trained on billions of articles from the web, much of it inaccurate.
But if you could marry their incredible language capabilities with a really tightly constrained set of information — by building a custom bot that only accesses a small set of documents — what could you accomplish? A lot.
I built three bots: One, to investigate how a newsroom might be better able to leverage the wealth of information contained in its reporters’ notebooks; two, to explore a more interactive user interface and story form; and three, to see if there was a better way to get technical or arcane expertise to resource-strapped newsrooms, especially on deadline. It took just a day and a half to get pretty decent — although far from perfect — results.
In this article:
Know More
The first experiment was simple: Collect the half-dozen scoops that Semafor’s Liz Hoffman has broken on investment firm 777 Partners and build a bot that answers questions only based on those stories. The idea was to mimic a newsroom tool for reporters that need to get quick summaries of the work their colleagues are working on.
Sure, they could read all the stories themselves (or call Liz), but why not just query a bot for specific information they need? The stories don’t list a CEO for the company, it had a good sense of what I was looking for here:
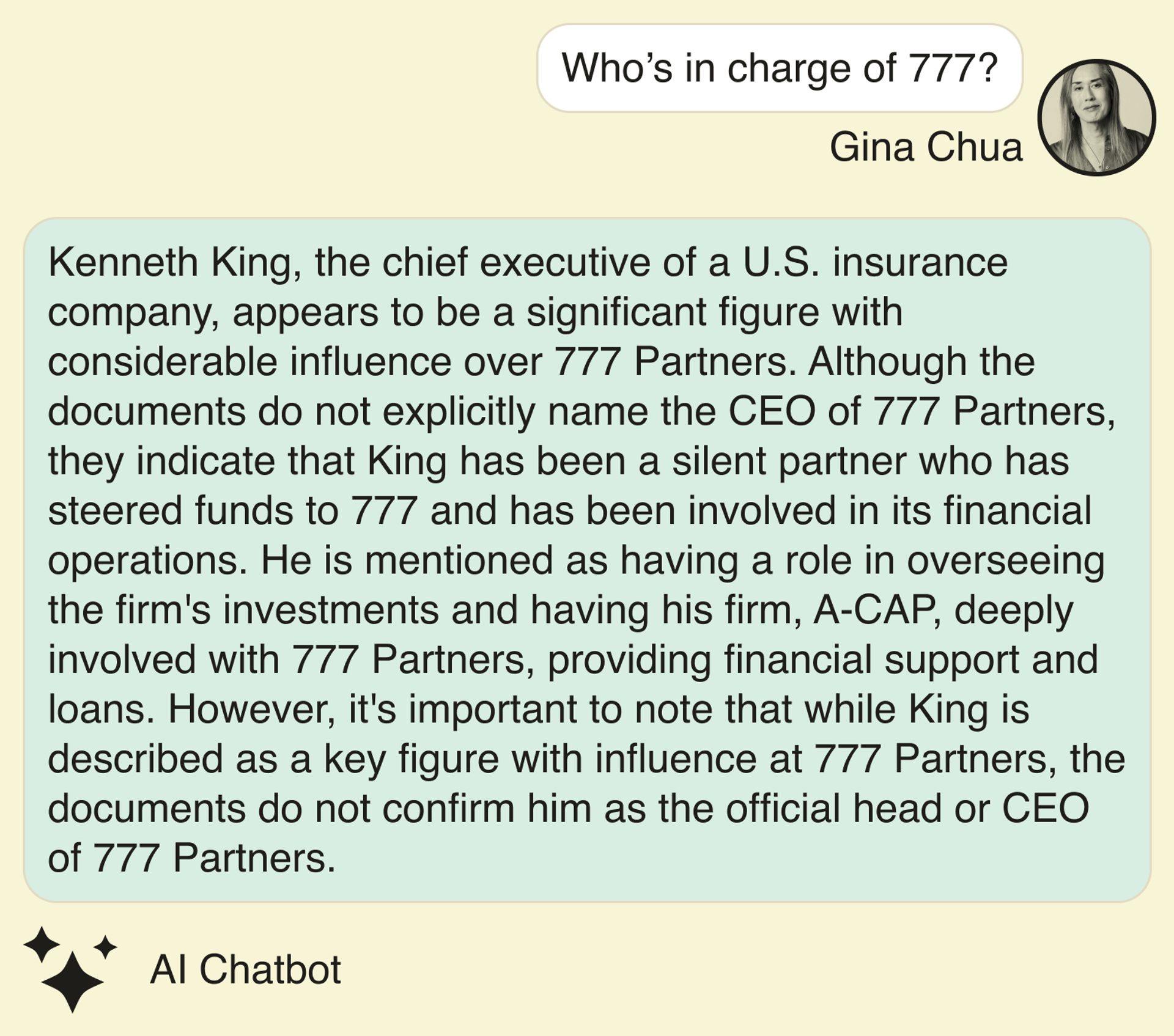
You don’t have to take the bot’s word for it; it offers citations that deep link to the source documents. That makes it an impressive search engine that “understands” context.
Imagine building a system across a newsroom that allowed reporters and editors to rummage through their published stories — or notes. Sure, you’d want them to double-check anything the bot told them; but you expect they’d do that from a Google search or if they read it in Wikipedia, too. This just helps them be more efficient.
The second experiment was to see if I could make a bot that would mimic a human conversation about a news story — an Alexa or Siri for news, but with much more capability. I took the same set of 777 Partners stories, but added prompts that asked it to answer as if it was talking to an acquaintance over a meal. (I tried asking it to talk as if it was conversing with a friend over a drink, but the language got too salty too quickly.)
The output was pretty good: It stuck to the facts, but in a much more casual tone — and most importantly — just gave me the information I needed when I asked, “what’s up with 777 Partners?” It spit out a quick summary of what had transpired over the stories so far; I asked whether any activity was illegal, and it told me about various probes into the company, as well as a denial from 777 Partners. I threw it a curveball by asking if I should worry about my investment portfolio, and it replied:
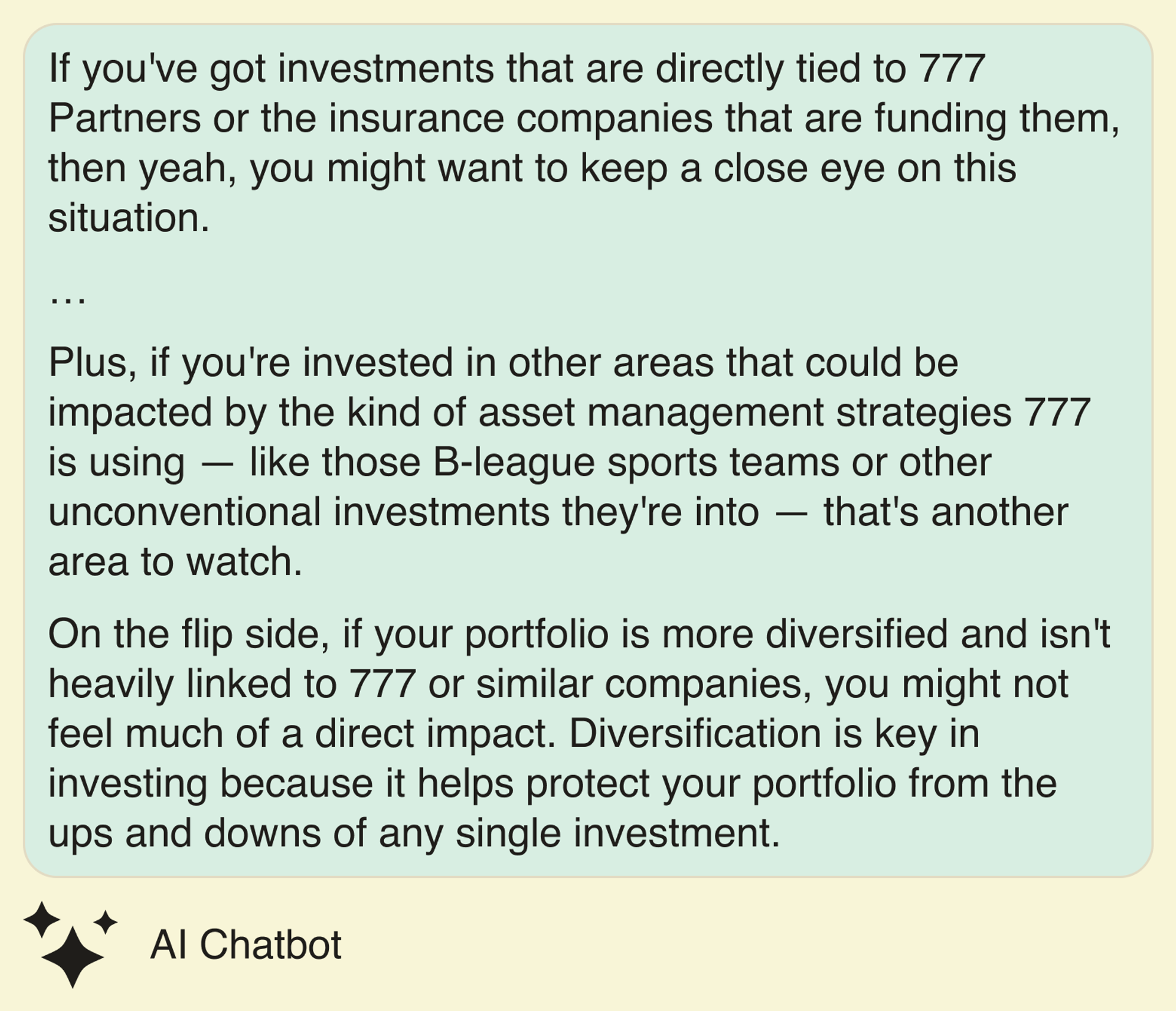
Which isn’t bad, even if none of that was in Liz’s pieces. That information certainly wouldn’t have explicitly been in any regular story, but it may in fact be the main angle a reader is concerned about. Why shouldn’t we find a way to let them explore that question?
The third experiment involved a completely different set of documents — the Trans Journalists Association style and coverage guide. (Digression: I’m on the board of the TJA, and I happen to think they have a really good set of guidelines for covering the increasingly fraught debate over trans issues.) Style guides are really helpful documents for newsrooms to ensure consistency of language as well as help journalists understand complex topics. (The AP, for example, has a great new style guide on covering AI.) The problem is that they’re usually long, few people read them in any detail, and good luck remembering all the nuances when you’re on deadline.
So I wanted to see if I could build a tool that could quickly offer the newsroom’s agreed-upon guidance to a reporter on deadline. For example:
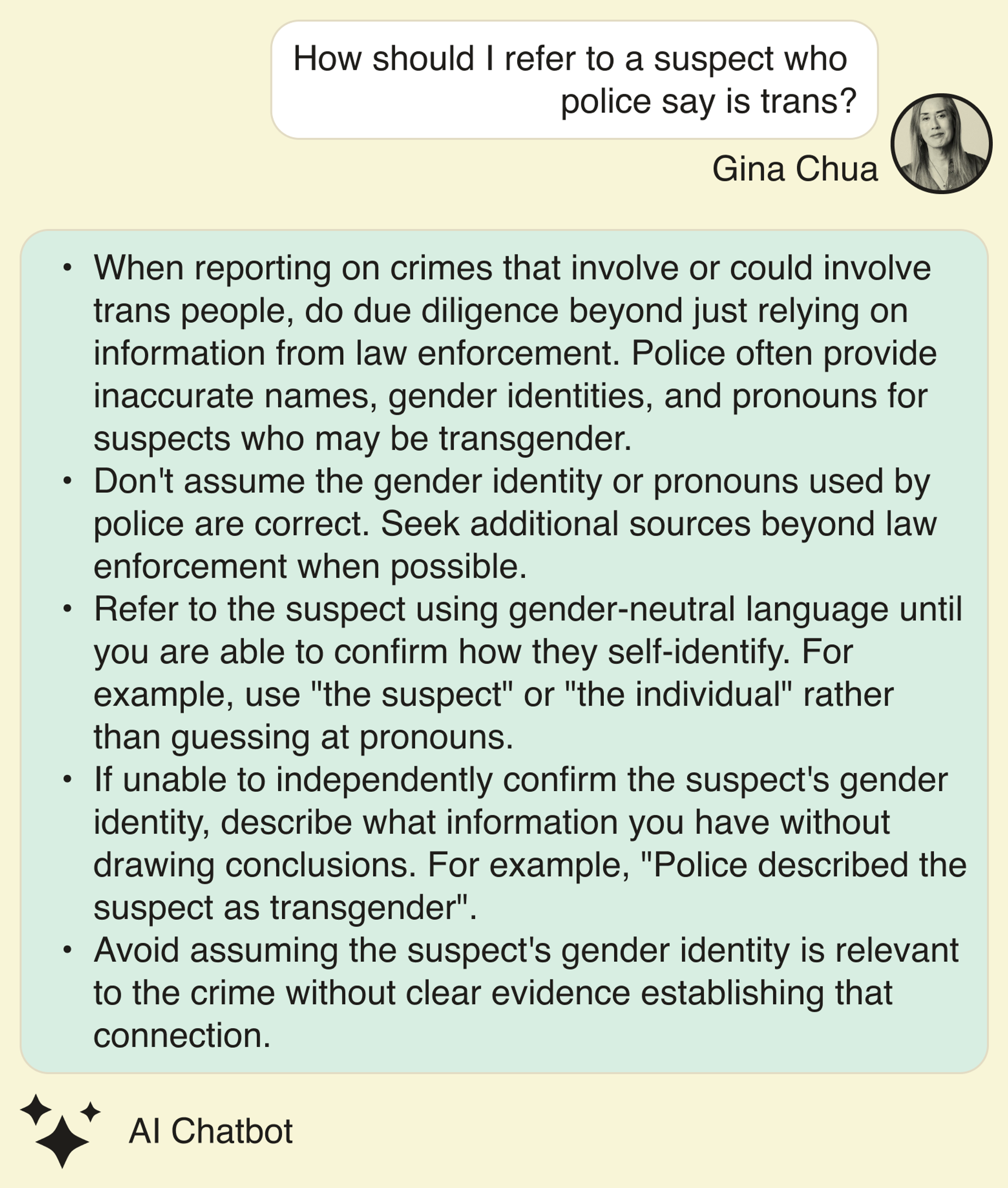
It’s not exactly what the TJA says, but the gist is pretty close. It handled more complex questions, such as how to refer to a source who doesn’t want to be identified as trans, and so on. And again, it provides citations so reporters can check the actual text.

Gina’s view
These are all pretty small steps, and pretty far from finished products. But then again, I built them in a weekend with nothing more than a subscription to Poe and basic English. I’m sure a real tech team would do a lot better.
Some of the potential uses — and upsides — are pretty obvious. Newsrooms are dense with information that people can’t find. I’ve followed Liz’s coverage of 777 Partners closely, but I’d be hard-pressed to quickly pull together a paragraph referencing that saga if I was writing a piece about a similar issue. Or, for that matter, if I was in a huge newsroom, I might not even have known about her scoops.
What custom chatbots offer is discovery on steroids; a way to query the collected — or at least published — wisdom of the newsroom, with some confidence the information presented has been verified. To be sure, it’s the sort of capability that Microsoft is rolling out for companies with its Office 365 Copilot program, but news organizations, with very specific needs for speed and accuracy, could use a really focused version of that.
Beyond that, the experiment with the TJA stylebook opens up a host of other intriguing possibilities. As the pace of news accelerates, and the size of newsrooms shrink, it’s harder and harder to bring deep expertise to bear when events break. A mass shooting, for example, isn’t something most newsrooms train to cover, yet when one happens, reporters are expected to master a wealth of information about weapons, laws and protocols, not to mention understanding best practices on how to deal with victims and their families, all on the fly. It’s not practical to be thumbing through a style guide when that happens.
But there are journalists and newsrooms who are deeply knowledgeable about such things. (The Trace, for example, is devoted to covering gun violence.) Why can’t we build custom bots for all those subjects, informed by specialists in those topics, that allow newsrooms around the world to query them when necessary? I know the TJA would love to be able to do that. (Send money! Please!)
And finally, I think we need to keep innovating around the story form. Semafor is already doing it with the Semaform, and a conversational chatbot is another interesting path to explore. Some journalists suggest that the best way to think about writing a story is to imagine you’re telling someone at a bar what happened; the custom chatbot puts that advice into practice.
The conventional story form — at this point more than a century old, and wedded to technology constraints as old — is built around the notion that the same piece needs to get to as many people as possible; chatbots offer us the possibility of a much more individual experience for users.

Room for Disagreement
As we all know by now, you can’t exactly rely on chatbots entirely. For instance, there’s just problem that the bots try to be too helpful. When I tested the one that offers advice on the TJA style guide with a non-relevant question about whether I should marry my partner, it first told me (correctly) that it wasn’t equipped to answer that question. Then it added:
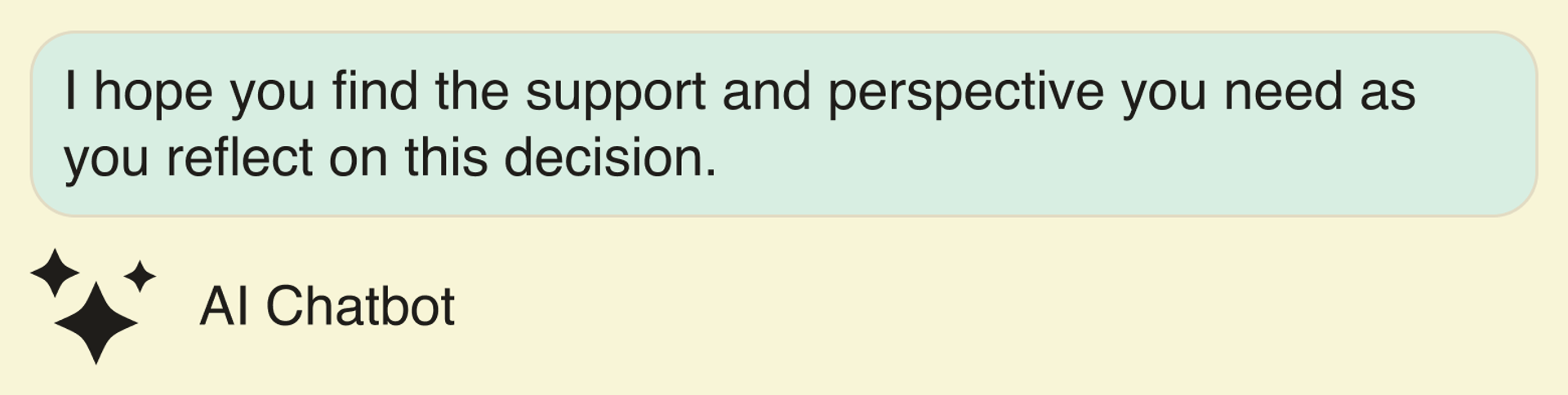
Thanks, mom.

Notable
- Casey Newton at the Platformer wrote about the copy editing bot he built.
- The New York Times examined the coming world of personalized bot agents and dangers and opportunities they bring.